Unlock the potential of solar energy with advanced machine learning predictions for optimal solar panel efficiency. Harness data-driven insights for a sustainable and efficient future.
Internationally, there is a rising trend of embracing renewable energy alternatives to mitigate the effects of climate change and promote sustainability. All solar power systems, in general terms, have become an integral part of this new global trend towards clean energy. However, with the introduction of technology, efficiency has been used as a parameter to achieve high-output production through solar panels.
Solar power systems that mainly consist of solar panels and the rest like inverters among others have become linked to sustainable energy services. Photovoltaic cells generate electricity from the sun, a source of free and replenishing energy. High-efficiency solar panels that convert sunlight into electrical energy play an important role in quantifying the viability of solar power systems. The higher the efficacy, the more energy output is generated and solar power becomes a better alternative to other sources including fossil fuel.
Prediction of Solar Panel Efficiency Using Machine Learning
The combination of machine learning and the solar cell industry is forming an unparalleled strength to define tomorrow’s renewable energy. The use of ML algorithms will later become a primary tool used in solving most problems, maximizing efficiency and thus allowing solar integration to be adopted by many.
Using machine learning, researchers at the UC Davis College of Engineering have innovated a unique approach to identifying materials for high-efficiency solar cells. The team’s approach uses high-throughput experiments and machine learning algorithms to reliably predict material behavior without a long experimental process.
Machine learning algorithms have demonstrated their effectiveness in predicting solar panel performance prediction and improvement as they could process huge amounts of data to derive patterns. The general idea behind these algorithms is to formulate models based on historical performance data concerning atmospheric conditions and other variables. Solar firms and scientists now can make decisions about selecting panels, locations, or system designs based on the above information.
Data is the heart of any ML or artificial intelligence algorithm, and predicting solar panel performance is not an exception. First, different data types are gathered such as historical performance information including weather conditions and maintenance documents. Pre-processing is done to preprocess the data and make it ready for training predictive models.
The chosen ML model is trained with a portion of the generated information. Training is intended to decrease this discrepancy as much as possible through parameter fine-tuning. A second part of the dataset is then used to validate by measuring performance, and if necessary, further refine model tuning.
Several ML algorithms are effective in forecasting the efficiency of solar panels. Normally, linear type or support vector regression models are employed to represent the relationships between input features and various levels of efficiency. Besides that, more complex models such as decision trees, random forests, and neural networks allow for representing non-linear relationships in the data.
Though solar panels produce electricity in daylight hours, energy storage solutions, such as batteries that allow the power supply to stay steady through periods of low sunlight or after dark are essential. Battery performance in solar systems can also be made better with machine learning algorithms. Predictive models enable predicting patterns of energy consumption, identifying the periods with elevated demand, and improving charging/discharging processes for batteries to promote efficiency in general.
In addition to estimating the efficacy of solar panels, ML and AI allow predictive maintenance. However, by analyzing maintenance event records and their impact on efficiency through the use of these technologies to predict that likely repairs are needed. This preventative measure minimizes downtime and optimizes yield.
This search for a more efficient solar panel has spawned different innovations in materials, design, and manufacturing processes. Machine learning algorithms contribute in part through this innovation cycle by analyzing different data sets to identify potential areas of improvement. Machine learning fastens the development of such innovative materials with enhanced light absorption efficiency, as well as futuristic surveillance systems to maintain desired alignment between panels.
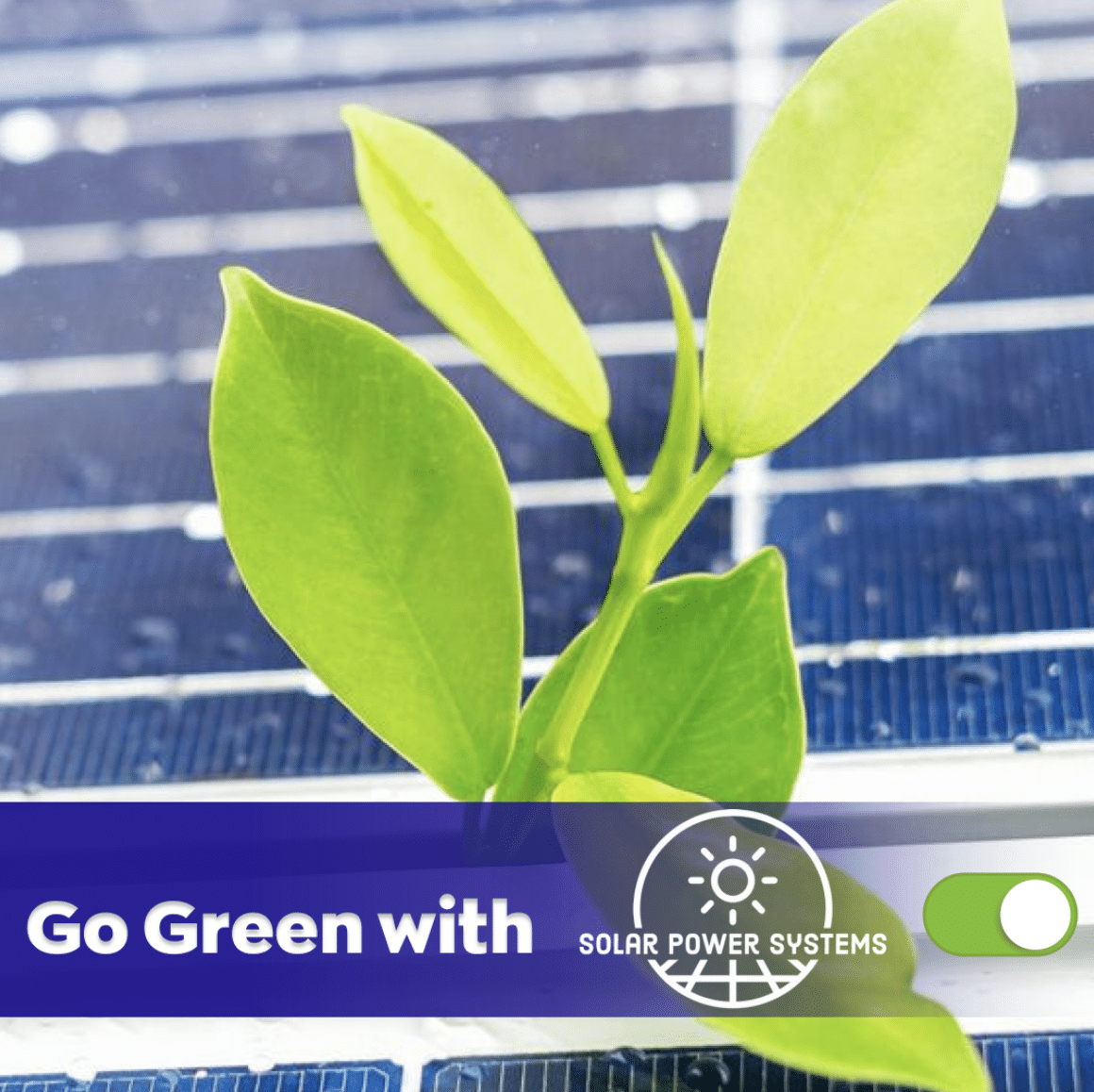
Some solar power companies have also gone into Machine Learning because they do not be left behind. For instance, the application of predictive maintenance algorithms is a good illustration. These algorithms can detect faults in live solar panel system feeds and their critical counterparts, thereby ensuring early warning of potential failures to avoid disuse. This system not only helps to keep systems integrity but also ensures affordable operation for solar companies.
Dealing with Challenges
However, as much potential there is in ML and AI that can be used to predict the efficiency of solar panels it comes with challenges. Researchers and engineers are actively addressing issues such as the quality, and quantity of data, model interpretation ability, and the volatile nature of environmental factors. Some of the major challenges faced by researchers and industrial professionals include access to high-quality data, and understandable models that are complex enough without being too complicated for interpretations; they also have constant adaption needs due to changing environmental conditions. On the other hand, these challenges also provide opportunities for more innovation and partnership in the solar energy sector. However, continuous improvements in the capabilities of ML algorithms and data availability methods increase model precision predictive ability.
With the progress of machine learning, predicting and optimizing solar panel efficiency by this technology will gain more precision. The clean energy future will be determined by solar systems, batteries, and innovations. As most of the solar panel manufacturing companies are planning to incorporate machine learning technologies, such have a competitive advantage over other players in this industry and can help them meet the increasing demand for clean energy with reliable and effective solutions.
To sum up, the evolution path towards a sustainable future for solar energy included developing machine learning. Solar systems are made more efficient by machine learning algorithms, which predict and evaluate collecting sunlight procedures. The joint venture of solar firms and machine learning professionals to develop a better scope of innovations will remain an integral index toward a brighter, cleaner future.