The purpose of data analysis is to help inform upcoming business decisions and identify strengths and weaknesses. Learn more about data analysis here.
Data analysis is a business analysis process that involves collecting and interpreting data. As organizations move to become more data-driven in the twenty-first century, data analysis is a tool of growing relevance. In this article, we'll look at the basics of data analysis, including what exactly it is, what types there are, and what the analysis process looks like.
What Is Data Analysis?
In business analysis, data analysis is the process of collecting, processing, and organizing data to draw meaningful conclusions. Data analysis can be used to evaluate past or upcoming business decisions based on real, empirical data, which makes it a valuable addition to any analyst's toolset.
Types of Data Analysis
There are dozens of types of data analysis depending on the nature of the data involved. However, no matter what data is involved, data analysis can be classified as one of three broad types:
Descriptive Analysis
Descriptive analysis is the process of analyzing data to describe what happened in a dataset. It does not make assumptions as to why something happened or what will happen in the future; instead, it reviews what really did happen.
For example, consider a dataset of the monthly revenues of a small coffee shop over the last 10 years. When conducting descriptive analysis, the goal is not to understand why the revenues were what they were or what they will be in the future, but to describe what the revenues were in the past. An example finding might be that revenues dropped over the last two years.
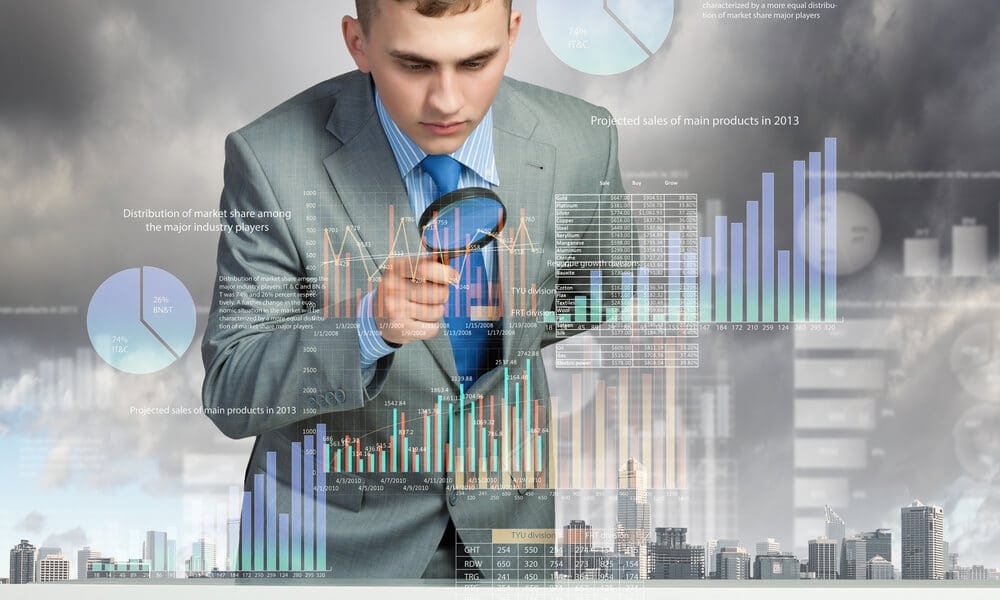
Diagnostic Analysis
Diagnostic analysis is a type of data analysis that involves diagnosing why things happened the way they did. It usually involves comparing two or more datasets, so as to identify any correlations, and potential causes of an event.
Let's continue with the example of the coffee shop, as used above. Thanks to descriptive analysis, the owner knows that revenues dropped over the last two years. Now, the owner wants to use descriptive analysis to find out why. To do so, the owner can compare the revenue trend with other variables, such as the trend in global coffee consumption or in the number of visitors to the shop. If a correlation is found, statistical techniques can be used to confirm these connections.
Predictive Analysis
Predictive analysis is when you use data analysis techniques to make predictions as to what will happen in the future. It usually takes into account findings from both descriptive and diagnostic analysis, so as to know what outcomes are commonly seen and what causes them.
In the coffee shop example, the owner knows how revenues have changed over the last 10 years and why. Now, the owner wants to know what revenues can be expected in the coming years. By analyzing the past trends and the variables that influence them, the owner can predict what will happen.
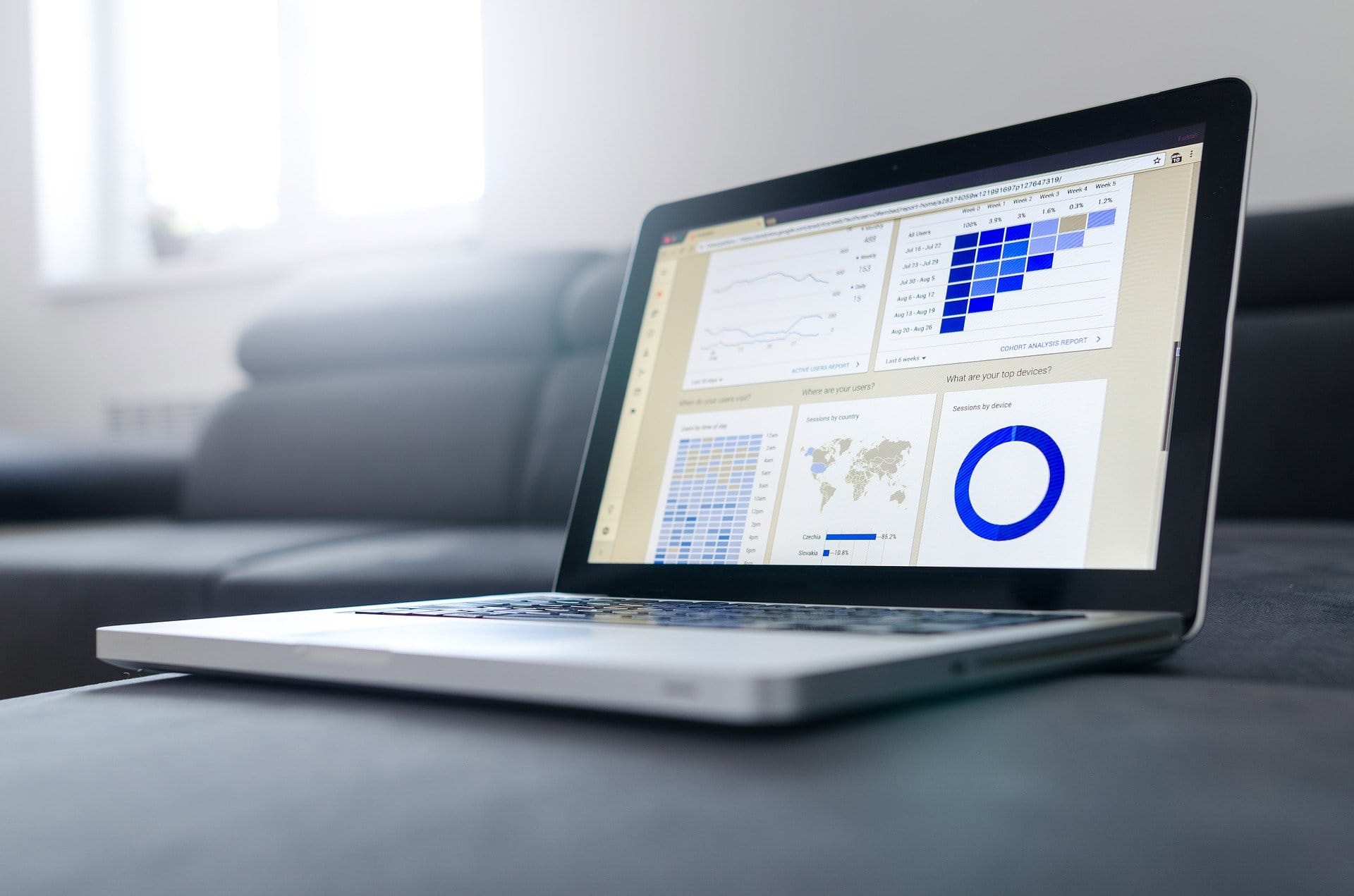
Data Analysis Process
There are a number of steps in the process of data analysis. You might be surprised to know that actually analyzing the data is a small part of analysis, since a large amount of effort must go into collecting, processing, and cleaning data so as to draw meaningful conclusions.
Data Collection
All data analysis starts with data collection. Generally speaking, data analysis works better with quantitative data, which can be represented numerically, than with qualitative data. As a result, data collection usually revolves around quantitative data, like the number or value of certain properties. This information can be collected from balance sheets (in the case of financial data), online analytics tools (such as Google Analytics), or even plain old counting (e.g. counting the number of people that order a certain beverage per week).
Data Processing
Once data has been collected, it needs to be processed into a usable format. Often, this means inserting the data into a database or spreadsheet, where it can undergo further analysis.
Data Cleaning
Following data processing, an important step in data analysis is data cleaning. This involves reviewing the dataset to ensure that all information is correctly formatted, and that there are no outlying data points which should be discounted.
Data Analysis
Only after data collection, processing, and cleaning can the dataset actually be analyzed. This is the step in which the analyst looks to draw conclusions from the data, either by reviewing the dataset manually or using statistical methods. In the case of diagnostic or predictive analysis, multiple datasets are combined to help understand what variables influence the dataset in question.
Why Do Data Analysis?
You may be wondering why you should bother with data analysis. After all, what is there to gain from looking at historical data? The answer is: a lot! Time and time again, it has been shown that historical patterns can inform what will happen in the future. In business, knowing what will happen in the future is of immense value, as it allows for smarter decision-making.
Looking at historical data may also be valuable for administrative reasons. For example, you can use data analysis to evaluate an employee's recent performance.
When to Do Data Analysis
In business, there are a few specific times at which you may choose to employ data analysis. Generally speaking, they include:
- Before making a big decision. If you're about to make an important business decision, you should use all the data available to you to help decide.
- For regular evaluation. You should also use data analysis on a regular basis, so as to identify what's working well in your business and what can be improved.
Final Thoughts
Data analysis is a multi-step process that's designed to draw meaningful conclusions from datasets. It involves collecting, processing, cleaning, and of course analyzing data. It can be used to describe what happened in the past (in the case of descriptive analysis), why something happened in the past (in the case of diagnostic analysis), and what might happen in the future (in the case of predictive analysis). In all three cases, the purpose of data analysis is to help inform upcoming business decisions and/or identify past strengths and weaknesses.